-- projects --
Lab/Design/Study Projects
The projects fall under one of the three categories: (1) Design Projects, (2) Lab Projects, or (3) Study Projects. Please fill the » Google form here « after selecting your projects below:
Design Projects
Project_WS2_2425_1_Design: Limitations of LLMs and Vision Languague Models in Video Understanding
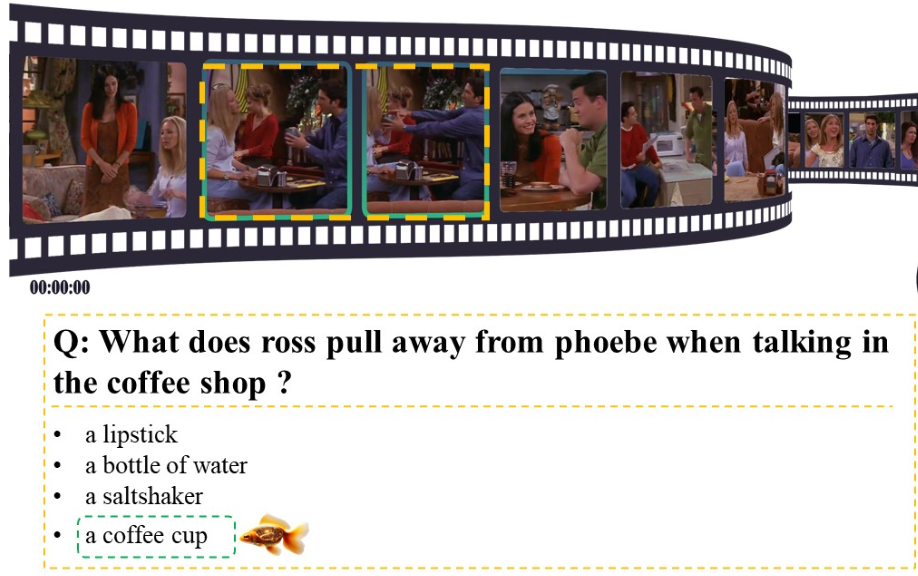
- This project is about validating effective prompting techniques to identify the limitations of Large Language and Vision-Language models in video event understanding. The task will involve developping (1) few-shot and k-shot promting techniques. (2) Testing the LLMs/VLMs response and understanding degree of Halucinartion in the response. e.g. MiniGPT-4. The main tasks in this project include :
- Develop inference pipeline using LLMs/VLMs (PyTorch-Lightning framework)
- Pre-processing and Post-Processing of Video and text Datasets
- Write a 8-10 page project report in LaTex (check Overleaf)
- Intermediate (15 mins) and final (30 mins) presentations of the results and overall project
Project_WS2_2425_2_Design: Multi-view Imaging and Rendering of 3D CAD Models
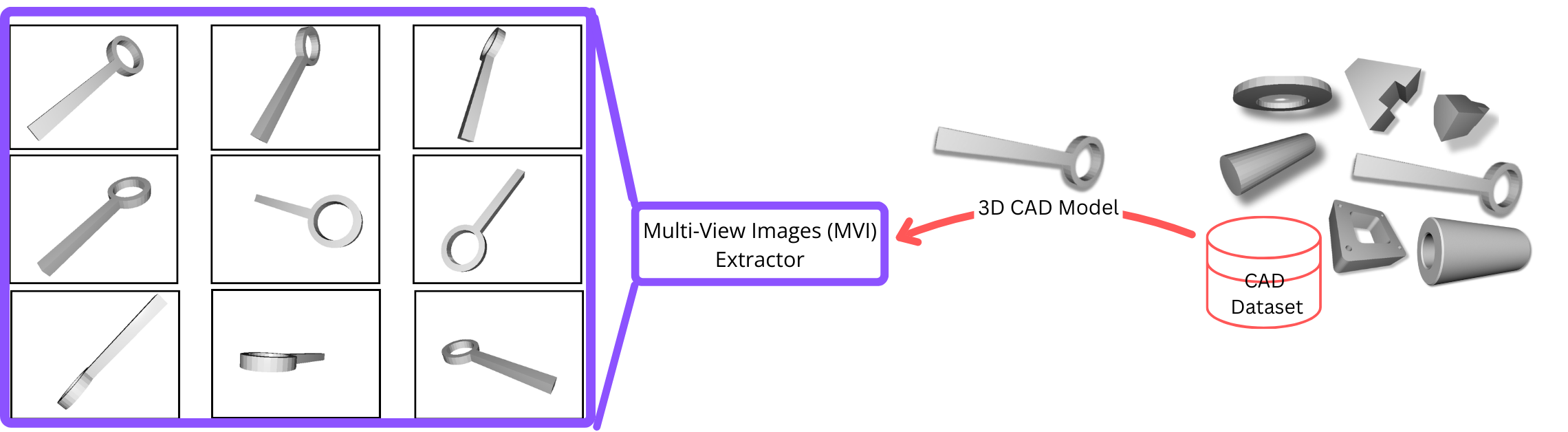
- This project is about development of precise multi-view imaging and rendering of CAD models such that inaccessible areas of such models are visible in the rendered images. Primarily, it is required to retrieve selective CAD model(s) from a data pool of CAD shapes along with their textual descriptions. e.g., user can input a text like "A CAD model of a disc with one hole at look like a key. Starting with a coordinate system setup, sketching the base and holes, scaling, transforming, and extruding to form a raised rim" to automatically fetch the 3D CAD model as shown above. Thereafter, a simulated multi-view imaging and redering tool will generate images of different visible parts. The main tasks in this project are:
- Implement a small protype of multi-view imaging and rendering of CAD models using Python packages like -- Open3D, Vispy,
- Implement an automatic CAD model's class category retrieval method
- Write a 5 page project report in LaTex (check Overleaf)
- Intermediate (15 mins) and final (30 mins) presentations of the results and overall project
Project_WS2_2425_3_Design: Vision-Language Models for Robust Visual Relations Recognition
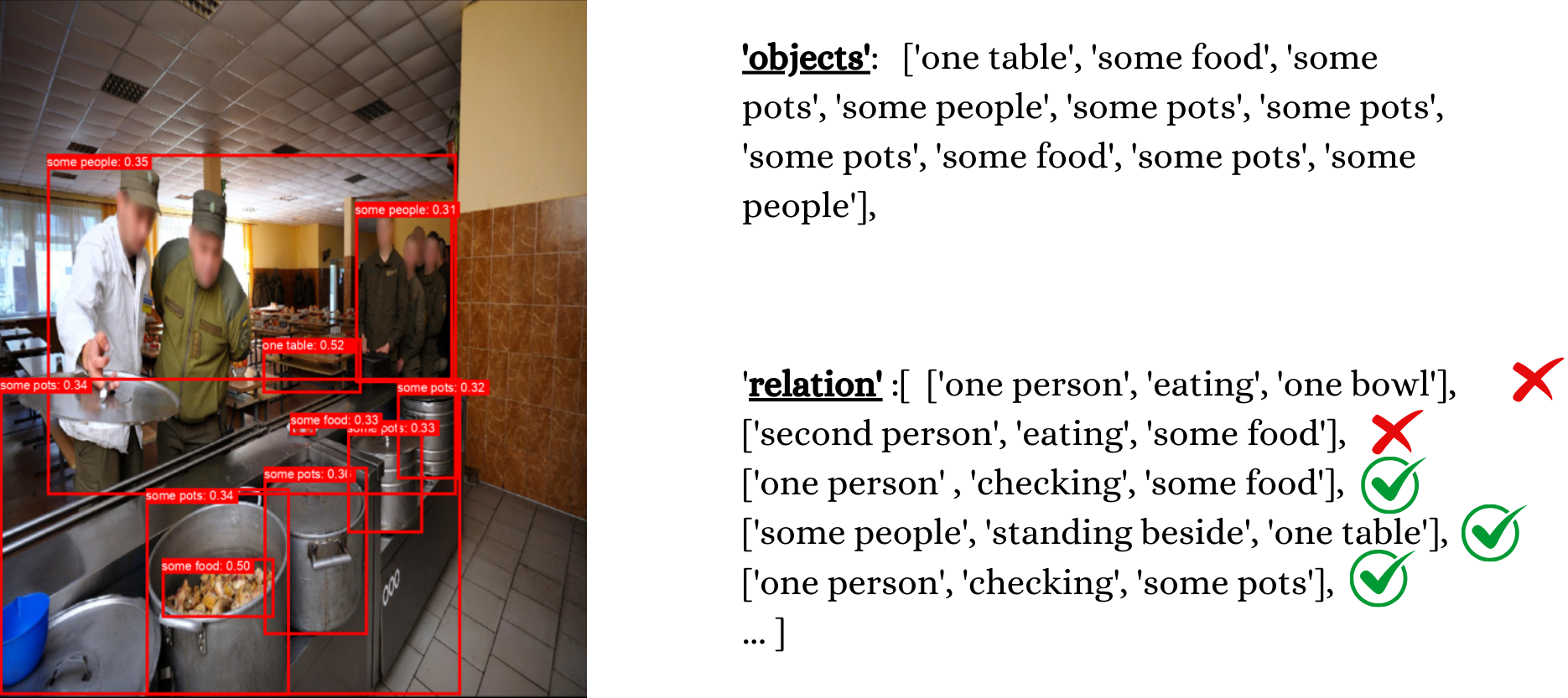
- Vision-language models have emerged as a powerful approach for robust visual relationship recognition, leveraging both visual and textual data to understand complex interactions between detected/grounded objects. These models may also integrate implicit visual grounding, enabling the identification and localization of objects within an image based on textual descriptions without explicit bounding box annotations. Notable works such as Scene Graph Generation by Iterative Message Passing (CVPR 2017), Vision-and-Language Navigation by Learning from Imagined Instructions (CVPR 2020), and ViLBERT highlight the progress in enhancing model robustness and grounding accuracy. The main tasks in this project include :
- Use state-of-the-art VLMs for visual relationship/motifs detection tasks and finetune it
- Generate large dataset containing positively and negatively meaning relationship triplets for a given image (see the figure above).
- Write a 5 page project report in LaTex (check Overleaf)
- Intermediate (15 mins) and final (30 mins) presentations of the results and overall project
Project_WS2_2425_4_Design: Vision Language Models for Robust Object Detection and Segmentation
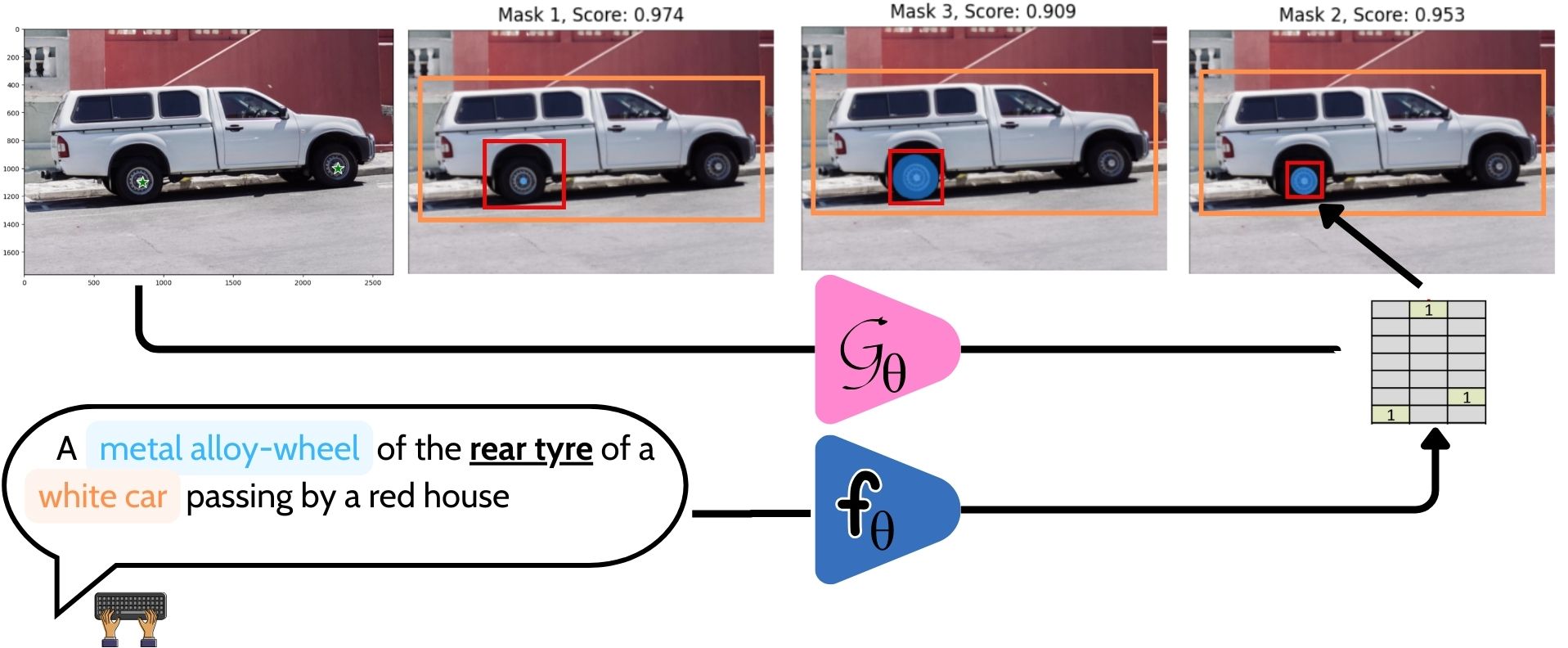
- This project is about funetuning existing Vision-Language Models (VLMs) for Robust Visual Relationship Recognition by integrating new type of visual and textual data. This textual data includes large annotations of (person/object)-to-(person/object) relationship triplets to enhance the understanding of interactions between objects in images. These models leverage implicit visual grounding, aligning visual entities with their corresponding textual descriptions without explicit annotations, thereby improving robustness and scalability. Key techniques involve cross-modal attention mechanisms and transformer architectures to capture complex relationships and context Li, Y., et al. TPAMI 2020. Recent advancements in VLMs have shown significant performance gains in benchmarks like Visual Genome, emphasizing their potential in real-world applications Zhang et al. CVPR 2021. Studies presented at conferences such as CVPR and ICCV highlight the importance of incorporating scene context and relational reasoning for more accurate visual relationship detection Zellers et al. CVPR 2021. The main tasks in this project include :
- Use state-of-the-art VLMs for visual recognition tasks and finetune it
- Implement Object detection, and Instance Segmentation tasks in a single deep multi-task learning frameworek
- Write a 5 page project report in LaTex (check Overleaf)
- Intermediate (15 mins) and final (30 mins) presentations of the results and overall project
Project_WS2_2425_5_Design: Python and Unreal Engine-based interface Design for Conversational LLMs
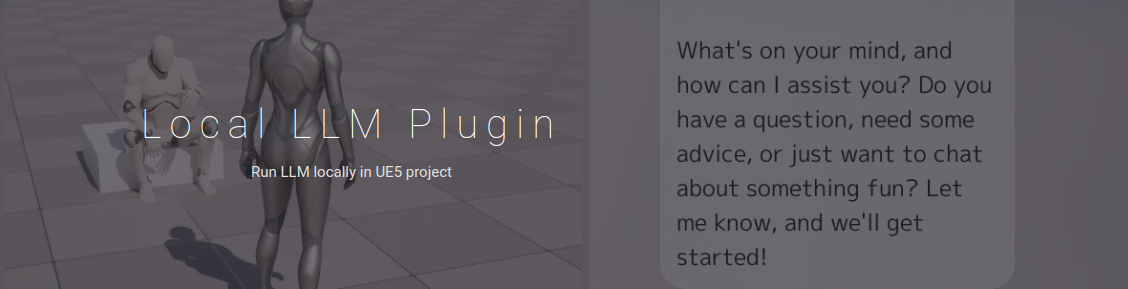
- This project is about developping an interface to plug LLMs and Unreal engine. The samples Graphical User Interface must have some basic functionalities -- e.g. (1) Automatic highlighting of interesting chat regions with different colors, (2) Automatic flushing of chat history beyond a specified context window, (3) Dynamic prompting based on slected parts of the previous contexts. (4) Automatic generation of muti-response from LLMs. The main tasks in this project include:
- Use state-of-the-art VLMs/LLMs as back end develop an interface where LLMs meet Unreal Engine.
- Python based user interface that integrates LLMs/VLMs and Unreal Engine/Unity
- Write a 5 page project report in LaTex (check Overleaf)
- Intermediate (15 mins) and final (30 mins) presentations of the results and overall project